. Introduction
Land use land cover (LULC) change is described as an ecosystemic transformation of existing land use mainly triggered by population rural–urban migration, which remains a complex phenomenon across developing cities like Nigeria (Adepoju, 2018; Sahana et al., 2018). These concerns on the ecosystem are escalated by the rising human population, resulting in increasing built-up territories over a stagnant small section of the global terrestrial surface (Bhat et al., 2017).
The LULC process evaluates the variation of the physical land surface properties, as it shifts from one LULC to another. This shift, if persistent over time, eventually triggers an LULC conversion that can be formulated for forecasting/prediction Joshi et al. (2016). The evaluation of territorial land use serves as an interface between human occupations and the ecosystem, especially as it affects planning policies for LULC mitigation (Silva et al., 2018). The changes in land use are dependent on the influence and significant impact of human-induced activities on the natural habitat (Rimal et al., 2018). The forecasting/prediction serves as an indicator for evaluating and comprehending the LULC processes and impending impacts for sustainable developments (Hossen et al., 2018).
Nigeria has been recording struggling cities with a rising population that continues to affect its territorial LULC transformations (Adepoju, 2018; Balogun et al., 2011; Bello et al., 2014; Oloukoi et al., 2014; Oluseyi, 2006). This greener pasture migration is a contributing factor to LULC changes. The challenge is triggered by unprecedented population increase, rising unemployment rate, increasing poverty levels, all putting pressure on the existing LULC in Nigerian cities (Nnaemeka-Okeke, 2016; Olujimi and Gbadamosi, 2007). Efforts to enforce regulation of LULC across Nigerian cities are embedded in the revised CAP L.5 Law of the Federation of Nigeria (LFN) of 2004 by policymakers. However, the relaxed attitude has resulted in the continued violation of the existing LULC variables (Nnaemeka-Okeke, 2016).
Over the years, the application of remotely sensed (RS) technology for LULC monitoring has been quite promising. The RS technology has become a vital instrument that is adapted to assist stakeholders to evaluate and pre-empt LULC as they occur over time. For developing cities with limited capabilities, advances in RS technologies are contributing to their substantial improvement in environmental resource monitoring-oriented researches (Aliyu and Botai, 2018b,a; Bello et al., 2014; Oloukoi et al., 2014). The findings obtained from the readily available RS datasets have continued to provide stakeholders with a better grasp and informed position for current and future policy plans (Aliyu and Botai, 2018a; Mahboob et al., 2015; Schaeffer et al., 2013).
Studies have utilised the available standardised RS datasets for temporal investigation of LULC across different developing regions of the globe (Bhat et al., 2017; Mubako et al., 2018; Rahman, 2016; Rimal et al., 2020). The accessibility to geoinformatics capabilities continues to ease the possibilities of researchers to analyse, model and predict LULC changes, especially over developing cities with limited data resources. The techniques utilised for LULC monitoring have helped in the design of various modelling algorithms such as linear regression (Hu and Lo, 2007), artificial neural networks (Maithani, 2009), multivariate regression (Al-sharif and Pradhan, 2016) and cellular automata (CA) / Markov chain (Mondal et al., 2017).
Studies have established that the present rate of LULC changes across Nigerian cities might result in a multitude of environmental problems, ranging from social segregation to environmental degradations. Anticipating short- or long-term consequences, this study hypothesised the effects of LULC changes for the city of Akure. The selection of this study area is based on the fact that it is the key link connecting the northern and eastern regions of Nigeria. Additionally, a past study has shown that the dense anthropogenic activity in the city continues to negatively affect the city's sustainable development. The rising expansion of Akure LULC is reported to be deteriorating as well as depleting available environmental natural resources (Balogun et al., 2011). To evaluate requirements of the current growth on existing Akure LULC as well as planning for future changes, there is the need for consistent evaluation of the city development for rational LULC change assessment. It is for this reason that this study aimed to conduct a post-1999 LULC analysis for the tropical rainforest city of Akure, Nigeria. This will be achieved via three objectives:
classify epochal land cover pattern,
determine land consumption rate (LCR) / land absorption coefficient (LAC),
project land cover over the next 20 years.
The manuscript is drafted to comprise of four sections. Section 1 presents the introduction. Section 2 describes that study area, datasets and methods. Section 3 presents the results with discussions, and Section 4 highlights the study conclusion.
. Materials and methods
Study area
The study area is Akure, the capital of Ondo State. It is situated in the south-west geopolitical zone of Nigeria (Figure 1). Akure comprises of two local government areas (LGAs), namely, Akure-North LGA and Akure-South LGA. The size of the study area is approximately 1,006.864 km2. The city has a population of 484,798 based on the population census that was conducted in the year 2006 with a fixed annual growth rate of 3.0% (NPC, 2010). Akure lies between the geographical coordinates of 7°3’40″–7°26’38″ north and 5°5’11″–5°29’39″ east. The study area is about 700 km southwest of Abuja (Nigeria's capital city) and 311 km north of Lagos State (Nigeria's megacity). The people of the city are predominantly from the Yorubas tribe. They are broadly classified into the following dialectical groups: Akoko, Owo, Ondo, Ikale, Ilaje and Akure. The residential districts are of varying density; some areas, such as Arakale, Ayedun Quarters, Ijoka and Oja-Oba, consist of over 200 persons per hectare, while areas such as Ijapo Estate, Alagbaka Estate, Avenue and Idofin have between 60 and 100 people per hectare. The annual temperature and relative humidity average are reported to be 25.2°C and 77.1%, respectively. Its vegetation is categorised as the tropical rainforest type, with the driest month being December. The major agricultural cash produce in the study area are cocoa and tobacco (Balogun et al., 2011).
Datasets
The following datasets were obtained for this study. A georeferenced (GCS Minna/Zone 31) administrative map of Akure was obtained from Nigeria's Office of the Surveyor-General of the Federation (OSGOF). The georeferenced administrative map of Akure was obtained to determine the extent of the study area from the Landsat image tile. Landsat 7 Enhanced Thematic Mapper Plus (ETM+) images of the year 1999 and 2009, Landsat 8 Operational Land Imager (OLI) image of the year 2019 datasets, all 30 m spatial resolution, were obtained from the United States Geological Survey (USGS) EarthExplorer website. The study datasets were adopted to evaluate the LULC change over a 20-year study period using a 10-year interval. The initial intention was to evaluate at the five-year interval, but there were no data for years 2004 and 2014 based on the data criteria adopted for the study. The imageries used were selected by adopting the additional criteria (cloud cover < 20%). The timestamp for the three Landsat datasets coincides with the Harmattan (dry) season in the study area. The reason for the adoption of the dry season is to control the datasets for exaggeration of vegetation that might arise as a result of consistent rainfall. The Landsat path and row for Akure are (190, 55). This parameter was utilised to identify and download the required from the URL (http://earthexplorer.usgs.gov). The Landsat imageries were downloaded to evaluate the LULC, change detection and future prediction of Akure, Nigeria. The medium-resolution Landsat dataset was adopted based on its free historic archive and open data policy. The Landsat data have demonstrated synchronised relevance in terms of processing and dissemination with ground systems (Zhu et al., 2019). The timestamps for Landsat images utilised for the study are 13 December 1999, 22 November 2009 and 10 January 2019 (as can be observed on the scene identifier in Table 1). For the change detection analysis, the supervised classification technique was adopted via the maximum likelihood algorithm (MLA). The valid supervised classification MLA technique has been broadly employed to analyse LULC changes (Sahana et al., 2018). To achieve the task, the following software was utilised: the Erdas Imagine v9.2 was adopted for band combination adjustment, layer stacking, supervised classification and accuracy assessment. The ArcGIS v10.3 was adopted for the gap filling of the 2009 Landsat image, clipping of study area/layout presentation. The IDRISI Taiga v16.0 was utilised for LULC change detection and future prediction.
Methodology
The methodology adopted for the study is illustrated in Figure 2. A detailed explanation of study area clipping, strip-line gap filling, atmospheric correction, supervised classification, accuracy assessment and change detection procedures are stated below.
Gap filling of strip lines
The producer georeferenced Landsat 7 ETM+ imagery for the year 2009 was downloaded with strip lines. The strip lines are as a result of the failed scan line corrector attributed to Landsat 7 ETM+ datasets obtained after 31 May 2003. The Landsat (LE71900552009326ASN00) data attribute indicated a scan gap interpolation of two for the 2009 imagery. A total of 28 strip lines were identified, accounting for 11.7% of the demarcated study area. The strip lines were gap-filled using the ArcGIS 10.3 software. This was achieved with the Landsat toolbox ‘fix Landsat 7 scan line error’. The procedure was performed for each band 1–8 before the atmospheric correction procedure.
Atmospheric/geometric correction
The atmospheric correction for the Landsat dataset was performed using the following steps. The digital number (DN) of each band was first converted to radiance at the level of top-of-atmosphere (TOA) radiance. The TOA radiance was then converted to TOA reflectance. The procedure was achieved using the raster calculator in the ArcGIS environment. The relevant metadata required for the procedure were obtained from the downloaded MTL text file described by Chavez (1996). For possible geometric distortions, the Landsat scenes utilised for this study were within the prescribed image-to-image tolerances of ≤12 m geometric root mean square error (RMSE) (Table 1).
Supervised image classification
Supervised classification was performed by developing a spectral signature of the verified land-use classes based on the Anderson (1976) classification regime. An accuracy assessment was performed to evaluate the conformity between the benchmark expected to be correct and the classified image pixels of unknown quality. Landsat 7 ETM+ and Landsat 8 OLI data were used to derive the LULC types for the study period using the Erdas Imagine 9.2 software. Before the supervised image classification, a false colour composite (FCC) analysis was performed to augment the ground-truth LULC information. This was achieved using the bands 4, 3, 2 in Landsat 7 ETM+ and bands 5, 4, 3 in Landsat 8 OLI. The five classes utilised are as follows: bare land, built-up area, cultivated land, forest cover and grassland. Bareland is any area with exposed land surface or rock outcrops. Built-up area is any area with asphalt or concrete roads. Cultivated land refers to an area under cultivation and intensive irrigation of crops pavements, buildings or houses. Forest cover is an area of dense tree cover with a thick closed canopy. Grassland is any non-vegetated, uncultivated farmland and open space. The rule-based feature extraction method combined with ground-truthed information was adopted for this purpose. The MLA was adopted for the supervised classification. It adopts the Bayesian decision rule based on the probability that a pixel belongs to a particular class. It is one of the most adopted and validated methods of classification in remote sensing (Hossen et al., 2018; Sahana et al., 2018).
Accuracy assessment
The accuracy assessment is performed to evaluate the degree of misclassification among LULCs to the user classified image from collected ground (training) data with the producer information. A total of 181 training data samples were obtained as reference samples over the study area for the individual Landsat images. Two methods of assessment were utilised to evaluate the accuracy of the classification procedure; the error (confusion) matrix and Kappa statistics (Table 2).
Table 2
Accuracy assessment of LULC for the study period
Change detection analysis
Change detection entails a timely and accurate assessment of the changes in the LULC variables at periodic intervals. In this study, change detection analysis was performed for the five LULC classes at intervals, 1999–2009, 2009–2019 and 1999–2019. Three change maps were produced to display the change of the classified images. The change detection statistics were performed using the IDRISI software.
The LAC and LCR was determined using equations (1) and (2) (Yeates and Garner, 1976). To compute the LCR/LAC, the following variables are required. They include the land area of the city (built-up) in hectares and the corresponding population size, for the respective years of interest. The estimated built area of the city for the epoch of interest was obtained using the classification distribution (Table 3).
where A1 is the extent (in hectares) for the early year, A2 is the extent (in hectares) for the later year, P1 is the population figure for the early year; P2 is the population figure for the later year.The population size was estimated (Parker, 2002) using the projected population equation (equation (3)) with the establishment census data of 2006 and the 3.0% growth rate for the study area.
The built-up area class is the key determinant for evaluating urban area growth. To formulate future urban area growth for the study area, the CA Markov model was adopted to capture the inherent spatio-temporal relationship based on the classified Landsat images. The CA Markov model analysis adopts the preceding LULC to project future spatial distribution (Liping et al., 2018).
The CA Markov model is one of the commonly used models used for monitoring the stability of land-use developments via future prediction. The model integrates CA with the Markov chain to predict the LULC trends and characteristics over time (Hamad et al., 2018). Equations (4) and (5) illustrates the computation of predicted land use (Kumar et al., 2014).
where S(t) is the system status at the time t, S(t+1) is the system status at the time of t+1; Aij is the transition probability matrix computed using equation (5). where 0 ≤ Aij ≤ 1 determines the probability of a pixel changing to another LULC or maintaining its original LULC.The CA Markov module embedded on the IDRISI Software (Sang et al., 2011) was utilised to generate the LULC prediction map for the year 2039.
. Results and discussions
Classification accuracy
An error (confusion) matrix was produced using cross-tabulation for the identified land-cover classes (Table 2). The matrix identified similarities between the producer accuracy and user accuracy to inaccuracies during the supervised classification process. The overall accuracy of the supervised classification was obtained to be 92.27% for the year 1999, 89.50% for the year 2009 and 93.92% for the year 2019. This accuracy was within the acceptable limits (> 75%). Additionally, the Kappa statistics recorded are 0.89 for the year 1999, 0.84 for the year 2009 and 0.91 for the year 2019. KHisto and KLoc accuracy are also indicated in Table 2.
LULC distribution
The distribution pattern of the changes in the spatio-temporal LULC in Akure is displayed in Figure 3. The figure showed an increasing proportion trend for the built-up land for the study period. This development inversely affected the proportion of bare land and grassland land covers for the period. This can be to a steady 3% growth rate for the study area, as reported by the National Population Commission (2010). Figures 3a–3c revealed the LULC configuration of the study classified images for the year 1999, 2009 and 2019, respectively.
Further analysis showed that bare land covered the largest chunk of Akure in terms of percentage area for the year 1999. It is followed by the grassland cover. The land-use gainer over the study period (1999–2019) is built up with 48.61% from 1.98%. This output inversely correlates with the depleting territory of the bare land cover (50.97% to 8.66%) and grassland (36.33% to 17.94%) from 1999 to 2019, respectively (Table 3). The rising trend of the built-up area class can be observed in Figure 3. These impacts have incited competition to create an avenue for accelerated acquisition of land area based on population developments.
Table 3
Temporal change/percentage of land cover classes for the study period
LULC change detection
Gains and losses
The gains/losses, net changes and contributors are presented in Figure 4. In Figures 4a–4c, the green bar indicates the gain per class in km2, while the loss (to the left) of each class is displayed the purple.
Figure 4
Change variation of land use/land cover classes in Akure. (a–c) gains (in green) and losses (in purple), (d–f) net variation (km2) in land use land cover (in purple) and (g–i) contributing the land use/cover to the net variation (km2) in built-up area for the study period (in purple)
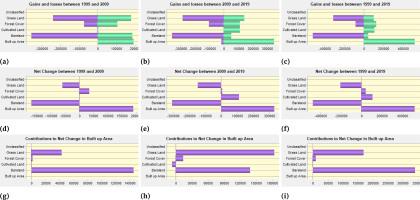
In Figure 4a, it can be observed that for the period 1999–2009, bare land class lost 329.63 km2 and gained 196.29 km2, built-up area class entirely gained 143.73 km2, cultivated land class lost 1.24 km2 and gained 12.13 km2, forest cover class lost 62.29 km2 and gained 94.87 km2 and grassland cover class lost 238.49 km2 and gained 184.64 km2. In Figure 4b, the gain and loss of LULC from 2009 to 2019 we derived to be bare land lost 351.15 km2 and gained 58.47 km2, the built-up area lost 19.95 km2 and gained 345.74 km2, cultivated land entirely gained 89.65 km2, forest cover lost 103.59 km2 and gained 112.21 km2 and grassland cover lost 265.66 km2 and gained 134.28 km2. The loss and gain from 1999–2019 (Figure 4c) showed that bare land lost 474.21 km2 and gained 48.19 km2, the built-up area gained 469.51 km2, cultivated land entirely gained 100.53 km2, forest cover gained 167.54 km2 but lost 126.34 km2. Grassland cover lost 278.15 km2 and gained 92.93 km2. The steady increase in the forest cover can be attributed to climatic variables as well as the population's cultural belief against forest intrusion.
Figures 4d–4f illustrates the net changes in terms of loss (negative) and gain (positive) along the x-axis for the five LULCs of interest between 1999 and 2009 (Figure 4d), 2009 and 2019 (Figure 4e) and 1999 and 2019 (Figure 4f). For the respective intervals, ‘bare land’ recorded −133.34 km2, −292.68 km2 and −426.02 km2; ‘built-up area’ recorded +143.73 km2, +325.79 km2 and +469.51 km2; ‘cultivated land’ recorded +10.89 km2, +89.65 km2 and +100.53 km2; ‘forest cover’ recorded +32.58 km2, +8.62 km2 and +41.20km2 and ‘grassland’ recorded −53.85 km2, −131.38 km2 and −185.22 km2. Figures 4g–4i identified bare land and grassland as the main contributors to the consistent increase in the built-up area class across the study intervals.
Land absorption coefficient/land consumption rate
The projected population estimates for the year 1999, 2009 and 2019 were computed as 394,176, 529,752 and 711,942, respectively (National Population Commission, 2010). Adopting the projected population size for the LCR/LAC analysis, it can be observed that the LCRs for the year 1999, 2009 and 2019 were computed (in hectares) to be 0.00505, 0.00362 and 0.0687, respectively. These values revealed there is a steady rise of the land consumed as a result of projected population growth. The LAC was also determined. With equation (2), the computed result revealed an LAC of 0.1635 from the years 2009 to 2019. Also, the absorption coefficient for 1999–2019 was computed to be 0.127. These LAC values obtained were higher than the coefficients reported by Salghuna et al. (2018) for Andhra Pradesh in India.
Urban area projection
Before the prediction, a genetic optimisation from the year 1999 to the year 2019 was performed. The 2009 image was used to validate the prediction of the CA Markov by comparing the predicted LULC in 2009 and the actual supervised classification for the year 2009. The overall Kappa coefficient was derived to be 0.864. This value was within the acceptable limits (Kappa index of agreement); thus, the prediction simulation was embarked upon. The CA Markovian analysis predicted the changes that are likely to occur over the next two decades (2039). It projected that about 675.011km2 of the study will be built-up area (Figure 5). The predicted built-up area increase (+37.92%) for this study was within limit when compared with the +27.31% increase for Damman coastal city, Saudi Arabia (Rahman et al., 2017). By contrast, the predicted built-up area increase was far less than the +111.34% for the Tarai region, Nepal (Rimal et al., 2020), and the +300% for Dhaka, Bangladesh (Ahmed et al., 2013).
From Figure 5, it can be deduced that the rising uncoordinated urban growth in Akure is very likely to translate to declined biodiversity and increased urban air pollution from increased, unregulated anthropogenic activities. This is, therefore, likely to expose the residents to respiratory health challenges as well as directly influence the city's urban heat island (UHI) analysis.
. Conclusions
This study adopted a validated procedure for evaluating the spatio-temporal pattern of LULC variables in Akure, Nigeria. The study datasets were selected for two decades (1999–2019). The pattern of LULC changes was established for the purpose validation and future prediction to the year 2039. The study result displayed significant changes (increase and decrease) of the LULC variables assessed for the study. The study has identified a periodic increase with the built-up area class. The studied LULC results showed that the net percentage change for bare land, built-up area, cultivated land, forest cover and grassland over the study period (1999–2019) to be −292.68 km2, +325.79 km2, +88.65 km2, +8.62km2 and −131.38 km2, respectively. The study analysis also revealed that the anticipated percentage change in built-up area from the year 2019 to 2039 (projected) will increase by 37.92%. The percentage increase of the predicted built-up area is within range when compared to other cities across the globe. The findings of the study serve as feasibility, thus will be beneficial to Nigerian policymakers who must begin to mitigate unregulated urban expansion.
It is worth noting the limitation of the study. Due to unavailability of datasets, the following factors were not taken into consideration. They include climatic, geophysical and socio-economic. The adoption of a medium-resolution satellite image (Landsat 2009) exposed scan-line error, MLA for the supervised classification and LAC/LCR analysis dependent on population projection (due to the unavailability of recent population census data across Nigeria) could cause differences of simulated results/predictions. Further investigation could consider other classification algorithms such as the random forest or support vector machine, as well as examine indicators for the rapid urban expansion. Within the identified limitations, the validated procedure adopted for this study was within the range of result with comparative studies, thus will expedite the mitigating capabilities for policymakers to address the challenges identified with the present Akure LULC. The findings of this study will guide future planning for rational LULC evaluation.